A Basic Framework for Growth Stocks and Industries.
Tools for dealing with high multiples, uncertainty, and qualitative data.
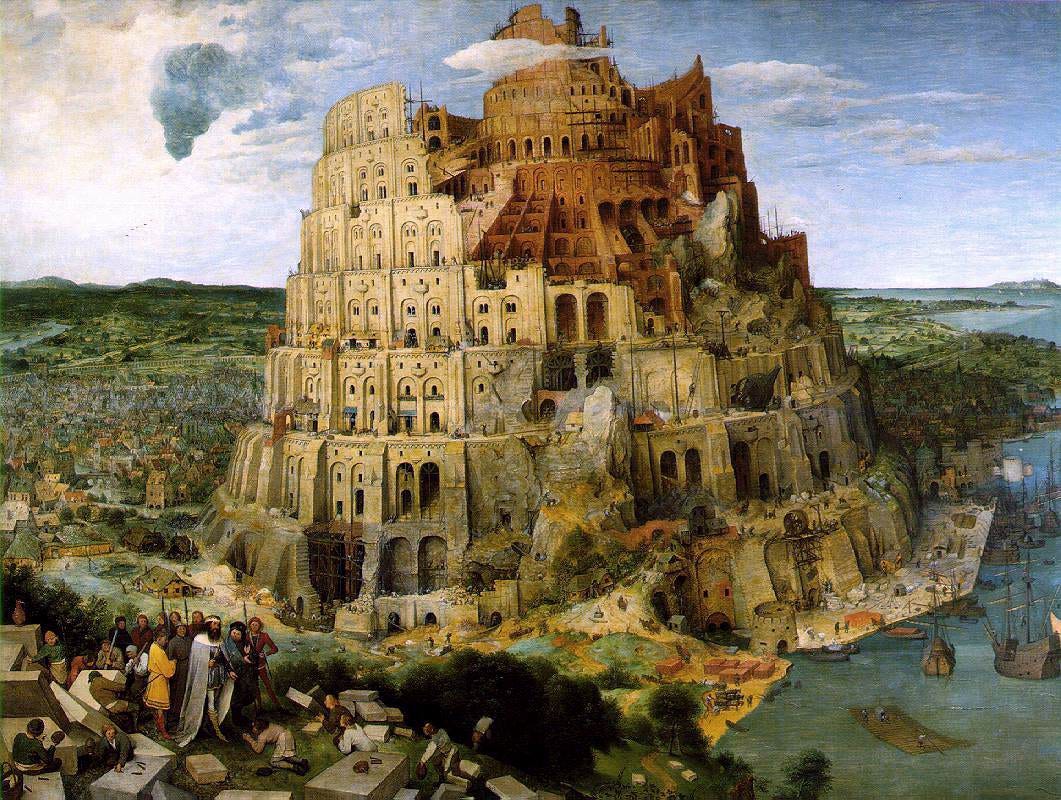
Growth stocks can offer tremendous value, especially at times of market stress. These companies give shareholders the chance to dream of achieving truly multi-bagger returns. However, growth investing is also way more dangerous compared to mature-company investing. Growth industries and companies are winners, prestigious, touted by the press, and interesting. This attracts high attention, higher stock prices, and more competition. Growth is a space where hype can easily build up, and any degree of rationality disappear, leaving the investor with a sentiment-based ticking bomb. Growth also requires dealing with an enormous amount of uncertainty and thinking about a dynamic, faraway future that is not fully anchored in today’s reality.
Growth, with both its opportunity and risk, requires a different mindset. This article humbly offers a framework for thinking about growth stock investing. It deals with two main aspects that I believe are the core of what differentiates growth from mature investing (always from a value perspective):
Valuations are future-weighted: The enormous majority of the value generated by a growth stock lies in the future. Based on current profitability metrics, the stock may screen as ultra-expensive or outright unprofitable. However, looking at the future, a high-multiple growth stock can also be cheap. Learning to apply a simple napkin model suited for growth companies can help us separate what is interesting value from over-inflated hype. This includes setting a correct hurdle rate, and estimating potential TAMs, growth rates, and margins. We also need to redefine the margin of safety and safety of capital concepts to the portfolio level to accommodate for the inherent risk of growth stocks.
We need to deal with lots of uncertainty: The future is uncharted territory, and we must deal with complex uncertainties. This is true for all companies, but especially so for growth. The technological, competitive, and execution landscapes are dynamic. The fact that the valuation has to stretch into the future adds a lot of compound volatility. Furthermore, growth companies are fundamented on intangibles that are hard to find in the financial statements. In order to deal with this uncertainty, tools like question mapping, research techniques based on intangibles, well-known competition models, and historical paradigms are very useful.
At the end of the article, I have added two appendixes with useful questions for understanding growth companies, and useful methods to gather qualitative data.
Each aspect—especially those in the models, paradigms, and expectations section—could easily be an article of its own. This piece focuses on presenting the general framework, tying these elements together into a cohesive structure.
Quipus Capital reached its first year of age! The blog now has 22 articles (industry primers, investment frameworks, and company deep-dives. You can check them all in the Index) and close to 600 subscribers across platforms.
I’ve updated the About page so you can get to know me better.
If you are new, subscribe!
And if you’ve been reading Quipus for a while, please complete a 7 question survey. It will help me understand what you like and don’t like about the content.
Portfolio and valuation in a growth environment
This type of investing is not for the faint of heart; it requires the vision to imagine the possible, to embrace and nurture the improbable, but with the discrimination to eliminate the impossible, and to prioritize the improbables with the largest upside - Vinod Khosla
For investors trained in the value investing school, constructing a portfolio of growth companies and thinking about their valuation can be unintuitive. Growth companies have high valuation multiples, low or inexistent yields, and they do not meet the minimum thresholds of capital preservation. We need to think differently about these aspects in order to understand value in the context of growth.
The margin of safety, options, and the power law
When dealing with highly uncertain futures, there is no way around the fact that we will be plain wrong many times. Some things will work very differently than we expected them to. This reality conflicts with two very important value investment tenets: safety of capital and margin of safety.
In value investing, downside protection is very important (‘The first rule of investing is not to lose money and the second rule is not to forget the first rule’ misquoted from Buffett). The safety of capital tenet advises to avoid investments with a moderate possibility of losing a big portion of the original capital. Meanwhile, the margin of safety tenet says that we should only invest in securities that provide a margin above capital protection so that the possibility of ever generating a loss is very remote.
The problem is that if those principles are followed to the letter, there is no way to invest in growth companies. If a growth company fails to maintain its growth, its share price will certainly collapse. Requiring absolute certainty that a company will achieve the necessary growth to meet our required return makes investment impossible, as such outcomes are fundamentally unknowable.
On the other hand, when expectations are met, the stock can produce a wonderful return over time —assuming those returns were incorporated in the valuation, as we’ll explore. These gains can more than compensate for losses in other names. In this sense, growth stocks are like options: some of them will be worthless (or worth very little), while others will be worth a lot when the final outcome is revealed.
This situation, where a few generate most, while others generate little or nothing, is known as a power law. The power law is the rule behind every instance of ‘1% of the population owns 50% of the wealth’ or ‘20% of the customers make up 80% of the profits’. Situations with uncertainty tend to exhibit this type of probability. The more into growth territory you go, the more this happens. Out of the public markets and into very early-stage companies, VCs have been found to generate 90% of returns from 10% of their investments.
Therefore, in growth investing, we cannot ask for security-specific capital protection. Instead, we should focus on portfolio-level protection. By conducting meticulous research and applying a sufficiently high hurdle rate in our valuation, we can ensure that our expectations are supported by solid fundamentals. On average, the investments should make money, even if some (or even if the majority) do not. A good research, analysis, and selection process serves as the margin of safety in growth investing.
This is not a free pass to take any risk without consideration. The growth investor's willingness to embrace risk can easily become the reckless, casino-like behavior that is so common in growth stocks. The process must be sound, the price has to be right, and the expectations have to be realistic, but we have to accommodate the fact that even with all that, sometimes individual investments will go south.
Differences and similarities with the VC approach
When thinking about growth, and particularly tech investment, it is natural to look to VCs for answers. They deal with much more uncertainty, backing companies without a working product or with really little revenue. However, the VC and public investor approach (our topic) to growth companies differ in key areas.
First, the VC is highly involved in the company's management, helping with hires, networking, and introducing customers. The VC, therefore, reduces risk by taking things under control, something most public investors can’t do.
Second, the VC has insider knowledge from the get-go. Being a private negotiation market, the VC can ask as many questions as he/she wants to during due diligence and then has a direct line with the company to ask questions as time passes. In contrast, public investors only access the information the company makes public and usually cannot even participate in open earning calls, not to mention closed ones.
These two differences reduce a great deal of uncertainty for the VC compared to the public investor. The VC has more agency on the outcome. On the other hand, the growth stocks of the public markets are much more mature than at the VC stage, having revenues, a working product, a proven market, and potentially even profits.
Despite the differences, we can learn some things from VCs, too.
First, we need to think about options and the power law, accepting that there will be mistakes but hoping to compensate them with successes. Second, we should ask for a sufficient return on all investments so that the uncertainty and risk taken are compensated, as we’ll explore in the next section. Third, we can learn the models and paradigms VCs use to think about markets and companies and project the future using very little, soft, qualitative data. We’ll delve deeper into these in the last section.
Finally, VCs think in terms of stages. They think about a company’s long-term future but focus much more on what is needed to get it to the next point, two to five years in the future. For a startup, these stages might be creating a prototype (proving the technology), getting the first 100 customers, crossing a revenue threshold, etc. As public investors, we can also use this approach and focus our attention on the next 5 years of the company, not the next 20.
Using a napkin model to make explicit the valuation assumptions
It is better to be roughly right than precisely wrong - J.M. Keynes.
Stocks are discounted expectations about the future. This is particularly true for growth stocks, which trade at high valuations compared to their current operations. In order to develop an understanding of whether or not the price of a growth stock makes sense, it is useful to explicitly lay down the expectations we have for the business. For this, a simple model is very useful.
I am not a big fan of elaborated quantitative models for companies. I believe that mathematical precision can hide the natural, irremovable underlying uncertainty that is part of these human affairs. That’s why the model I propose for growth companies is very simple, with only four components: a hurdle rate, a growth rate series, an operating margin series, and an exit multiple. This approach will allow us to determine if a stock price is attractive or too elevated based on the expectations needed to generate a specific return.
The hurdle rate
Any present value model has a discount rate that converts future inflows into present value. The discount rate is extremely important in growth companies because the inflows can be very far away, meaning their discounted present value is incredibly affected by the discount rate. For example, $100 in 10 years at 10% is worth $38 today, versus $16 at a 20% discount.
I refer to it as a “hurdle” rather than a discount rate because it is an input determined by the investor. Therefore, it serves as a hurdle that the company (or, conversely, the stock price) must overcome. The hurdle rate represents the return the investor expects from the investment.
Many investors (incorrectly, in my view) derive the discount rate from the market. For example, they might use CAPM or some peer cost of equity model. The problem with this approach is that the investor lets others (the market, which is prone to optimism and pessimism cycles) decide the fair return. If someone is happy with a 5% return, does that mean that I should be happy to pay $62 for the promise of a $100 return in 10 years? I beg to differ. I want 20%, and if I don’t get that (the price is $16), I am happy not investing.
So, what determines the hurdle rate? The investor's own beliefs about risk and about a fair return for that risk. We can think of risk and return as a staircase. The risk-free return of the US Treasury currently sits at about 5%. My view (and this is extremely subjective) is that a fair equity return for a pretty safe and quality company is 12%. If 12% is fair, then I want more, maybe 15% or more, for it to be an opportunity. A growth company is necessarily more risky than a quality, developed company, and therefore, I think an attractive return is closer to 20%, at a minimum. However, this is for each to decide.
Asking for high hurdle rates on any investment is normal, but particularly so for growth. According to Reaction Wheel, citing KPMG, VCs backing companies about to IPO (bridge to IPO financing) still ask for 25-35% returns. A post-IPO growth company is safer than a pre-IPO company (more access to capital, daily liquidity), but using a 20/25% hurdle rate is still reasonable, IMHO.
The second factor is whether or not the hurdle rate should be the same for all companies. Theoretically, the more risk there is around the cash flows we expect, the higher the rate. The problem is that estimating this risk is not easy. We cannot determine if the cash flows we project are more certain for company A versus B because both are based on sparse qualitative data and many assumptions. For example, say you invest in two names, both pass a 20% hurdle rate ON PAPER (this is the important part), but at the end of 5 years, one did generate the 20% return, and the other made a 0% return (or worse, a loss). Then, the portfolio of these two risky companies returned 10%, below the hurdle. Does that mean we should ask for a 40% return to each name, speculating that 50% will generate a zero return?
Unfortunately, this is an art and not a science. Sometimes, you will feel that the evidence is very strong and be happy with a 20% return because you think that is what you will get. Sometimes, the evidence is not as good, and a higher return might be needed.
A tangent: return crystallization
The relationship between risk and return is clear in finance: higher risk, higher return, lower present value of future cash flows (lower stock price). The opposite is also true: lower return, lower risk, higher present value of future cash flows (higher stock price).
The idea that risk decreases, leading to a rise in stock price, is absolutely fundamental to growth investing. In fact, I would dare say that most of the returns in growth investing (from VC to public markets) come not from projected growth itself (which can be projected from the get go) but rather from a decrease in the risk or uncertainty tied to that growth.
This has an important implication for investors: since present (PV) and future values (FV), discounted at a specific rate, are equivalent, buying a stock at a high price (or, conversely, a low hurdle rate), means we are recognizing that the future cash flows are more certain in the present, from the seller’s perspective. For the seller, those cash flows are now more certain (they got the PV side of the equation, the price of the stock), while we, as the buyer, now carry the risk (the FV side of the equation) in exchange for a potentially mediocre return.
I call this situation ‘return crystallization’ because the seller (who bought at high risk and sold at low risk) saw the uncertain future ‘crystallize’ into a certain outcome, a higher PV and stock price. As investors in growth stocks we should never buy future cash flows as certain, crystallizing the return for others. On the contrary, we should buy future cash flows as highly uncertain (heavily discounted, high hurdle rate, low stock price), and see the return crystallize in our hands as the outcomes become more certain over time.
Exit multiples
A PV model requires cash inflows to the investor. Dividends would be the classic format, but growth companies generally only pay dividends very late in their life, and decreasingly so because of tax reasons.
Therefore, the model requires an exit sale at some point in the future. This introduces a big problem: estimating how much someone would pay for future earnings (or sales if the company does not generate earnings).
Similar to the hurdle rate, one popular approach is using market comparables or peer averages. However, I do not like this approach because market optimism/pessimism can change on a whim, and the comparable multiples we thought about, disappear. Rather, I prefer to use the multiple I would be willing to pay for the company in the future. Because I consider myself a conservative investor, I think the multiple I would pay is an arm’s length, fair multiple.
Something important to consider for the exit multiple forecast is that growth companies suffer from multiple contraction. As they grow, their future growth prospects tend to decrease. Their value gets closer to the present, and the multiple on earnings shrinks. Therefore, it may not be accurate to estimate that the multiple will simply ‘remain the same’ 5 or 10 years in the future. A recent example of this is Amazon doubling its EBITDA between 2021 and 2024, but its stock price remaining flat because the EV/EBITDA multiple halved (see picture below).
One simple rule for a fair exit multiple is treating earnings (if they exist at that point) as a yield and adding growth to reach our desired hurdle rate. For example, if we ask for a 20% hurdle rate and we think the company will be growing at 10% CAGR in the future, then the fair multiple is 10x.
Using an exit also allows us to work in stages. While it’s important to consider the company's long-term prospects (such as how big it could get at maturity, in 10/15 years), we can focus on the path to the next 5 years. At the end of the day, pretending any form of certainty about what can happen to a company in 15 years is self-delusion.
The fundamentals: revenues and margins
The company needs to generate earnings to pass our hurdle rate. Therefore, our model will have two fundamental components: revenue growth and operating margin. Estimating these is the most critical portion of the research work and the investment decision process. It is so important that this article has a section dedicated to it (“Tools for dealing with uncertainty”).
As a summary of the process, we start with one question: what will earnings be in 5/10 years? From there, we branch out into several others: What will the market size be in 5 years? What will the company’s market share be in 5 years? What will the operating margin be in 5 years? This leads to an iterative process. We then answer some of those questions using both hard (numeric) and soft (qualitative) data, and project the future by combining this data with rules, models, paradigms, and our intuition.
Some thumb rules around hurdle rates
Modeling helps make the assumptions behind an investment explicit. This is necessary because the human brain is not very used to the mathematics of growth and compounding. Some of the results needed for a growth stock position to be profitable are unintuitive. Here are some examples:
If a stock maintains its multiple on earnings, then the earnings CAGR has to equal the hurdle rate (unless it pays dividends or buys back shares). This means if you want a 20% return on a stock, the stock has to grow earnings by at least 20% (compounded). This is already a high bar.
If we add multiple contraction, the growth rate requirement is even higher. The thumb rule is that the cumulative growth in earnings equals the fall in multiple (percentage-wise). For example, if the stock falls from a 20 to a 15 multiple in 5 years (-25%), we need an additional 25% of growth to compensate. If that happens over 5 years, then it’s about 4.5% additional growth per year. Over 10 years is 2% additional growth.
The real challenge of multiple contraction happens when the multiple falls a lot (say from 35x to 17x in Amazon above) because then you need a lot of growth just to compensate for that contraction.When one is not used to thinking about high hurdle rates, it is surprising how much the discount rate eats into very high growth rates and margins. As an example, below we have a company that quadrupled revenue in 10 years, going from a 20% negative margin to a 25% positive operating margin. The price to be paid today is barely above 1x sales for a 25% return. This is just the math of returns. This means most of the time we will need to ask for low prices for the investment to make sense.
Tools for dealing with uncertainty
Ad hoc, ad loc, and quid pro quo, so little time, so much to know - Jeremy Hillary Boob, PhD. - The Yellow Submarine
Typing numbers in a spreadsheet is easy. The napkin model above is only a tool to keep us in the ground, thinking about the cost of money. The actual hard work is thinking through the assumptions that go into the model.
The market size, the capacity to steal share or create the market, and the potential cost levers in the operating structure, are questions with very indefinite answers based on mostly qualitative data points. The next section deals with techniques for creating information with soft data in a context of very high uncertainty.
Mapping the questions
When we are building the thesis behind a name, there will be tons of questions: Is the company’s product the best? Is it improving faster than the competition? Are the larger players interested in this segment? Is management capable? Is engineering talent entering or leaving the company? How big is the market? Is the market growing? What is the CAC? Is it decreasing with scale?
All of these questions will feed the expectations we will use in our napkin model and, therefore, affect what we consider the fair price for a growth stock. However, not all of these questions are equally important, and not all of them can be answered in the same way. We need to map the questions to tackle the most important ones first. This consists of three parts: classifying, ranking, and branching.
Classifying the questions is the first step. Not all questions can be answered with the same type of data. Some questions (the most important generally) cannot be answered at all:
Hard-knowable: There is a definite, numeric, publicly available answer. Maybe the answer is on the financial statements or earnings calls. An example is the current market share and growth over time so far.
Soft-knowable: We can find an answer, but it will not be numeric. Rather, it will be based on several qualitative queues. Examples are quality of management, customer opinion, and position in the price scale.
Unknowable: most things that lie in the future are unknowable by definition (unless there is a contract, for example). In this area, we are speculating based on the knowable answers and the models (below). Examples are whether adoption will occur, if competition will increase, etc.
The next (or concurrent) step is to rank the questions based on how much they will contribute to our understanding of the company. This is not an easy task, but it is absolutely critical because otherwise one is simply blindly looking for data points, which can then introduce availability bias.
Unfortunately, the most important questions will generally be unknowable, mainly because we are dealing with the future. In that case, we need to move one level below and ask what are the drivers that will influence the unknowable but critical questions. Generally, these will be of the soft, qualitative type, which in turn might be influenced by some quantitative questions. In this way, we branch the questions in an inverted tree.
After this process, we will have an idea of how to structure the research process (what to look for), and also a map to understand where are the gaps in our understanding after some research. Below is a simple graphical example.
At the end of the article, Appendix I has a potential list of questions that will help us understand a company and think about its future (inspired by Michael Shearn's The Investment Checklist).
Finding soft-data
Most of what we can know will be in the form of soft data. One reason is that competitive assets are intangible and uncountable in most growth companies and, therefore, are not reflected in financial statements or public data directly. Another is that most of the important information is competitive-sensitive, meaning companies do not want to disclose it.
This means that we need routines to find and use soft data to understand a growth company's future. Mapping the questions above is the first step. We also need sources of soft data. Here are some examples (more in Appendix II):
Review websites and consulting firms: G2, Gartner, IDC, and Youtube channels.
Contacting customers and ex-employees via LinkedIn or calling if B2B (calling anywhere in the world is easy and cheap with VoIP options).
Visiting the websites of the companies, comparing the sales process. Pretending to be a customer.
These are time-intensive and only yield small tidbits of data after significant effort. For example, one may cold call or email several customers before anyone decides to answer. In addition, one must filter the data through potential biases, the most important of which is availability bias (the data we have access to is not representative of the population). For example, consulting firms tend to be positive on most products as they don’t want to tarnish the relationship with the companies, or the employees or customers we talk to might have special motives to talk (for example, being angry at the company), or forum writers being haters vs evangelists and not representing the silent middle ground.
Still, this is the best we have to project key questions into the future. In my experience, even small samples yield good results if they all point in the same direction and are well organized. Also, this is another reason why mapping is important: without a map indicating what is more relevant and without pre-planning for how to find the data, we will be simply finding data points at random and then trying to conclude something out of it. This introduces another bias, pattern-seeking bias (seeing patterns where there are none, like shapes in the clouds).
Using models and paradigms
We can only access a limited amount of data (both hard and soft). Most of that data will be qualitative queues, like some comments in an earnings call or one comment in a forum.
Translating that data into information and then projecting that information into speculation about the future requires soft functions that work with few observations. This is where the models and paradigms enter the picture. The models and paradigms will help us transform soft answers into unknowable answers. Even more, knowing models and paradigms will make us more attuned to finding queues in small, seemingly irrelevant details.
Models
A model (basically a rule but called a model for grandiosity) is simply a function used on qualitative data. It tells us that if qualitative condition A is met, then future outcome B is more likely. They can go from very broad and wide, to only a small set of cases. In fact, any time we learn a story from a business, it becomes its own small rule/model (A happened, then B happened) applicable to other cases.
There are many models, from the very large and broad (books dedicated to them) to the super small and specific (e.g., types of growth strategies for B2B SaaS companies).
Some of the most important models used to determine competitive advantage and unit economics in growth industries are:
Five forces: A company's competitive position results from its power (or lack of) against customers and suppliers, how easily the product can be replaced, how easily new competitors can enter or leave the industry, and how competitive the industry is.
Broad strategies: Cost (being the cheapest), differentiation (being the best), or niche (one of the two but in a small market).
Niche market dominance: it is very common for growth companies to start in a small market, which is ignored or uninteresting for larger competitors. If that is the case, competition is easier at the beginning but may become harder at later stages.
Network effects: a network becomes more valuable as more users participate, creating a self-reinforcing competitive advantage. A particular type of network is a platform or ecosystem (like an e-commerce website or an app store) where two sides can exchange value.
Returns to scale, experience, and cost leverage: most intangible-based companies have low marginal costs on those intangible assets, meaning their marginal cost structure becomes asymptotically cheaper as they grow.
Resource dominance: the company controls something that is very difficult for others to get or build (IP, employees, the network, etc.).
Adoption curve: technologies and products go through a process of adoption that first grows rapidly, then wanes, and then grows again (Chasm Model) or it may be first slow, then fast, and then plateau (S-Model).
Besides the large models, we also need many small models that apply only to specific industries, stages of company development, and areas. These include things like which channels are more valuable for a company with X type of product, what constraints a B2B SaaS company faces in finding engineering talent, or what the best ways to avoid tying capital to customers during growth are.
These small models allow us to speculate on qualitative queue A becoming outcome B. For example, I’m evaluating two B2B SaaS companies. I know both are targeting large companies. In a conference call, I read that company A’s preferred acquisition channel is paid marketing. I can mark that as a negative because that kind of channel works for much lower-friction products (say, buying a t-shirt online). On company B, I read that they focus a lot on a sales force and a specific chunk of the market. That is a positive because, in the past, that strategy worked much better.
Paradigms
The paradigms are the historical, technological, political, and market contexts in which the company is developing. Knowing about paradigms helps us match the model and the data with the appropriate context and, therefore, also provides an idea of where things might go.
For example, in IT history, there have been four or five changes in the computing paradigm (mainframe, PC, internet, mobile/cloud, potentially AI coming). When the paradigm changes, there is a big opportunity for market disruption (fertile ground for growth companies, bad for mature companies). Each change in this large technological paradigm has reverberations in subindustries. For example, service software to businesses moved from a license-based model (adapted to the PC era) to a variety of SaaS models (adapted to the mobile and cloud eras).
A bigger paradigm is the theory of technological revolutions (proposed and explained in detail by Carlota Perez), under which humanity went through three technological revolutions in the modern era: steam, mass production, and IT. These revolutions start very slowly, then accelerate, and finally tap (an S model).
According to the paradigm, the IT revolution started slowly in the 1940s, began to accelerate in the 1970s, and has only recently finished building its foundations (the internet, mobile, the cloud) to begin the final phase of application and maturity. We are, therefore, currently in the last stages of the IT revolution, meaning technological advancement in IT will moderate, and IT will no longer be a growth but a mature industry (like autos or banking). This paradigm explains the emergence of super-dominant IT players like MAGA, which are the standard in mature industries.
If Perez’ theory is correct, it has enormous implications for growth investing. For example, the explosive growth of the past is less likely to replicate in infrastructural products (like the PC for Microsoft, the smartphone for Apple) and much more likely to be repeated in small bursts in applications (like Uber, or the digital bank Nu). Further, and even more important, we might be at the early start of a different technological revolution (biotech, cheap energy). If that new industrial revolution has different capital requirements compared to the extremely capital-efficient IT companies, then we might have different financing, return and growth models altogether.
We can also think of a political paradigm. For example, the stage of globalization between the 1990s and 2018 during which most of the IT acceleration happened, compared to the current internal/external conflict period, may be more resemblant to the 1960-70s. We might even combine a political paradigm with a technological paradigm. For example, the era of high conflict between 1914-1945 was marked by the transition from steam to mass production and from UK to US hegemony. Could we repeat the same pattern now, with the transition from IT to something new, and the conflict between China and the US?
Finally, we have market-specific paradigms. An example is the difference between B2B SaaS companies and consumer software companies in terms of their competitive dynamics. In B2B SaaS, the customer needs are very specific, and the sales process is complex, creating lots of small niches, each one of which can sustain a big company (or even several in one niche). In consumer companies, the needs of the customers are less segmented (everyone uses Uber in the same way), with a very simple sales process, on which scale provides a big advantage. This has led to super-concentrated markets that span across segments.
Rules&Paradigms caveat
The process for collecting rules and paradigms is basically reading books, and blogs and listening to podcasts/interviews:
The canon of company strategy explores the classic models explained above in much more detail: Porter’s Competitive Strategy, Christensen’s Innovators Dilemma, Helmer’s 7 Powers, Thiel’s Zero to One, Cohen’s Cold Start Problem, Moore’s Crossing the Chasm, and others.
Some of the blogs I like are Ben Thompson’s Stratechery, Ben Evans, Brian Balfour, Paul Graham, Gerard Neumann, and Blair Reeves. There are dozens of free blogs, especially among VCs.
Colossus has a fantastic collection of growth and business-focused podcasts.
A caveat is in order, though. Like most theories in any discipline involving humans, the number of observations (n) is low and contaminated by biases like availability, survivorship, halo, etc. For every one of the large models and paradigms, one can easily find counter-examples that the authors did not consider. In fact, there might be no rules at all in growth and technology, but we might still find patterns that give us an illusion of knowledge (pattern-seeking bias). Things are easy to explain after they happen but more challenging to predict before they happen. We should not blindly act on the conviction that a rule will determine the future because if one thing is certain, is that the future is uncertain.
Final thoughts on the process
I don't look to jump over 7-foot bars: I look around for 1-foot bars that I can step over - Warren Buffett
Evaluating a stock price, determining the underlying expectations, and finding the data to back or reject those expectations is hard work. It also involves a lot of risk. For that reason, we need to be very disciplined only to buy when the conditions are in our favor and when the data we have gathered really backs our expectations. Most of the growth ideas will go into the ‘too hard pile,’ and that is ok. The goal is to make money, not to be the smartest.
When one is not used to thinking about high hurdle rates, it is surprising how much the discount rate eats into very high growth rates and margins. This means that maybe one cannot participate in growth stocks most of the time because the stock price already incorporates too much optimism (remember: optimism = lower risk = lower hurdle rate = higher stock price = lower return for the holder = higher return for the seller). This is fine. Discipline in only buying when the deck is stacked in our favor is paramount because we are dealing with highly uncertain situations, with high risk, and we are already accepting a high potential capital loss. If a stock is too expensive, we can just wait. Stocks become cheaper for idiosyncratic reasons all the time.
Capital cycles
Like all things in life, growth goes through cycles, albeit different from those studied in this blog related to mature industries. Whereas in more mature industries, the cycle shows up in revenues and margins, in growth industries, the propelling force of an expanding market somewhat dampens this business cycle.
In contrast, growth industries show marked capital availability cycles. At some points in the cycle, there is a lot of optimism, a lack of investment vehicles in the growth industries, plenty of capital, and, therefore, lofty valuations. At other points, there is more pessimism, more companies chasing capital, less capital willing to be invested, and therefore low valuations.
The capital cycle also happens at the company level, especially if the company is unprofitable after going public. If a growth company needs a capital infusion to reach the next stage, and that need matches a moment of relatively dry capital markets, then the valuation can get pretty low, compared to previous periods.
Alasdair Nairn, in his book ‘Engines that move markets,’ mentions five capital stages of a technology. Each stage has its own hype and pessimism cycle in the capital markets. To cross from one stage to the next, capital is needed, and it is at that point that stocks may become cheap:
Feasibility: the tech is not yet proven, this is where VCs and the government invest.
Prototype: very early market products, already at a scale sufficient to IPO or become large (but unprofitable companies). The industry starts to have specialized newsletters and the general public starts to participate.
Commercial viability: many large companies, heavy investment (particularly if capital intensive).
Refinancing, restructuring, and consolidation: not all public companies can survive in a more consolidated market.
Success: the survivors of the consolidation generally have open ground to continue growing into maturity.
Ending quote
Mentioning Nairn, his book is a great historical account of several hype and capital cycles in growth industries, albeit very concentrated on capital-intensive growth industries (channels, railroads, telegraph, telephone, internet infrastructure), which differ from the current intangible-heavy IT growth companies.
The book ends with a warning phrase that seems to summarize the challenges of growth investing:
‘Anyone who studies the historical record is more likely to come away with an appreciation of how little certainty, predictability or inevitability there is about technological advance translating into financial rewards (...) If by chance you had been able to identify the genius of Henry Ford at an early stage, it would have been important to wait until he had been bankrupt twice before investing in his third venture, the Ford Motor Company (...) For the personal computing age one would have had to wait for Apple to appear, but remembered to exit before Microsoft attacked the space; to understand the dynamics of IBM and the coming of Compaq, but be ready for the arrival of Dell! This would have meant ignoring hundreds of other competing companies that grabbed the headlines and market share during the early years. In the case of Apple, the investor would later have needed to decide when and how to buy back into the company’s shares in anticipation of the extraordinary success of the iPhone.’
Appendix I: question-list to draw a company map
When looking for information and gaining insights into a company, we need to avoid availability bias (giving more importance to what we know or have access to than to what we don’t know). As proposed in Michael Shearn's The Investment Checklist, a list of questions is very valuable for classifying the information we get and identifying gaps in our picture.
U: What is the revenue in 10 years?
U: What is the market size in 10 years?
S: How big is the market today?
M: How many customers are there
M: How much do they spend on average?
U: How much will the market grow?
U: How does future adoption look like?
S: What problem does the product solve?
S: What adoption model to use?
S: Are there switching costs into the product?
S: Is it easy and cheap to test the product?
S: Who makes the decision to adopt in the customer? (ex: CTO, developers, CFO, individual).
S: Does the category get better or cheaper over time?
S: Does category improvement drive more usage from the same customers?
S: Does category improvement drive more users?
S: Are customers permanent or do they tend to churn/rotate?
U: Is this a global or regional product?
S: Do local competitors have an advantage?
U: How much market share do they have in 10 years?
M: How much market share do they have today?
S: Are they creating or stealing market share?
S: How good is the product versus the competition?
H: How is retention rate?
F: Customer reviews
F: Consultant or technical reviews
M: How does the price compare with the competition?
S: What is the rate of improvement of quality and/or price, versus competitors?
S: Are there positive/negative loops in quality/price? ex: network or scale effects
S: What sources of optionality does the company have?
U: How rival will the competition be?
S: How rival is competition today?
S: What are the drivers of competition in today’s market?
S: Who is the closest competitor? Who is the largest competitor? How does the company compare with these two?
U: At which point do competitors go from creating to competing for market share?
U: What are the drivers of competition in tomorrow’s market? Which competitive model is applicable? Is there a winning strategy?
U: Are the largest players in the industry participating in the market?
S: Does the company control any strategic resources? Will it gain access to any resources?
ex: network, scale effects, IP, barriers to entry.
S: How good is the company at improving the product?
H: Absolute and percentage R&D.
H: Absolute and percentage number of R&D workers
S: Innovation pipeline (past and future) compared to competitors
S: Is the market cyclical?
S: What type of cycle? Margin, revenues?
S: What drives the cycle? Macro, idiosyncratic, supply?
U: What are the margins in 10 years?
S: What is the company's cost structure?
M: What is the marginal cost of the product?
M: What are the fixed costs of the operation?
S: Does the business have capital requirements?
M: What is the investment needed to gain a customer / enter a market?
M: What is the cost of acquisition of the client?
S: Do they need to repeat this cost over time or is one-time?
U: How will the cost structure change?
S: Are there returns to scale?
S: How leverageable are the fixed costs?
S: How long will the investment/market gain period last?
U: How much pricing power will they have?
S: How concentrated/diversified is their customer base?
S: Who has more power, customer or company?
S: How hard is the sale?
S: Can competitors fight for the same customers (see competition section)?
S: Do they have different segments with different pricing power?
S: What would happen if the company raised prices?
S: Is the product inherently commoditizable at some point in the future?
U: How good is management?
S: How has execution been so far?
S: Employees' opinions of the management team?
S: Do managers seem honest in their communications?
H: Have managers achieved what they set out as goals over time?
S: Have managers lowered the goalpost before?
S: How much do managers take responsibility versus blaming external factors (macro, competition, etc.)?
M: Are managers good stewards of money?
H: What are the largest expense buckets?
S: How efficient has management been on growing without expanding costs?
S: What is the quality of previous M&A and cap alloc decisions?
H: Do managers own stock?
S: What’s management compensation policy?
S: Does the company have different work processes, or team processes, than the competition?
Appendix II: Sources of soft-data
The process of obtaining soft data is time-intensive and laborious, but knowing where to look for is an important first step. Here is a list of potential sources and information-gathering techniques:
Comparing with the competition
Review websites and consulting firms: G2, Gartner, IDC.
Individual reviews: YouTube channels, specialized forums in Reddit or Discord. These websites are an open-source focus group for almost any consumer product. The amount of insight one can get here is hard to compare.
Contacting customers via LinkedIn or calling if B2B (calling anywhere in the world is easy and cheap with VoIP options). Available for companies with mid-sized or large customers (Youtube or forums are better for individual customers).
Industry-specific substacks or newsletters are fantastic sources of dispersed insight.
Visiting the companies' websites, comparing the sales process, and pretending to be a customer.
googling site:[companysite] will give you a much more structure way to navigate a website
Using or demoing the products if possible. Sometimes, investor relations might be able to stage a demo.
Constructing a product launch time-line from press releases or industry news sites.
Searching for senior-level management interviews in youtube with ‘[company name] CTO’ or similar. These managers might be more open to talk shop in an industry setting than the CEO on an earnings call.
Google map reviews can be useful for location-based companies like retail, restaurants, etc.
Industry
‘[industry name] primer’ in Google or Scribd.
Industry associations sometimes have great reports.
Industry news websites (like [industryName]dive.com) are not great for overall insights because they concentrate on the details and small news. Still, they are good resources for second-stage research and follow-up.
Management / company culture
Proxy filings: how much stock managers own, how they are compensated.
Glassdoor: comments from employees about working for the company.
Contacting ex-employees on Linkedin (you can search for previous company, specific positions, roles, etc.).
Usage metrics
Semrush or Similarweb: estimates webpage traffic over time based on Google rankings.
Google Trends: Estimates how a topic (company name, category name, product, etc.) trends in Google search, configurable by region and year.
Github: shows product adoption for software products
builtwith and stackshare: which pieces of software are used by the largest products. Important for software B2B products.
General
Reviewing earning calls. If time is limited, one can focus only on Q&As or investor days or search for keywords (margin, leverage, customer, demographic, adoption, share, competition, etc.).
Visiting ValueInvestorsClub to see if someone already made a deep dive. No need to reinvent the wheel. Similar to a company or ticker name search on Twitter or Substack.
Disclaimer: The opinions expressed in the Blog are for general informational purposes only and are not intended to provide specific advice or recommendations for any individual or on any specific security or investment product.
Not a word on stock-based compensation and the resulting dilutive impact?